Data to Intelligence: How AI Empowers RWD for Target Disease Selection
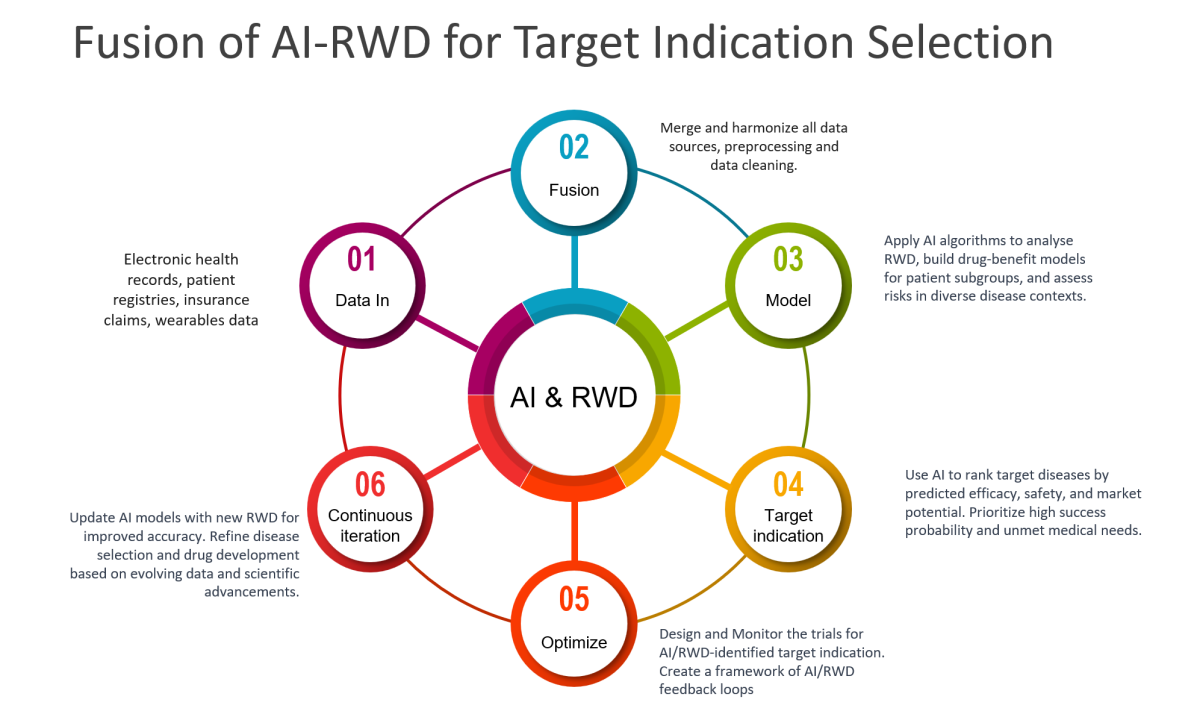
Introduction
Selecting target diseases is critical for biopharmaceutical companies, impacting drug development success and patient outcomes. Traditional methods, guided by key opinion leaders, literature reviews, omics analysis, randomized controlled trial (RCT) data, and competitor choices, can be subjective and suboptimal. Integrating real-world data (RWD) and artificial intelligence (AI), a comprehensive data-driven approach emerges as a transformative solution for more objective and efficient indication selection in the biopharma industry.
he Role of Real-World Data (RWD)
Real-world data (RWD) becomes a transformative force, providing biopharma companies with insights into approved drug performance in real-world scenarios. It goes beyond clinical trials, encompassing diverse patient populations and healthcare settings. RWD empowers companies by:
- Broadening drug performance understanding: RWD creates a comprehensive view of how drugs perform across demographics and disease contexts.
- Revealing benefits and risks: RWD uncovers unexpected outcomes or drawbacks associated with a drug, contributing to a holistic understanding of its real-world implications.
- Informing investment decisions: RWD from sources like electronic health records and patient registries helps companies make informed choices about prioritizing drug applications.
- RWD unlocks existing drug potential, supports superior treatments, and enables judicious healthcare decision-making.
Artificial Intelligence in Indication Selection
Artificial intelligence complements RWD by extracting insights from complex datasets. AI algorithms extrapolate findings from RWD to patients with different disease characteristics, enabling a more personalized approach to indication selection. This allows biopharma companies to identify patient subgroups that may benefit most from a drug, optimizing the development process and increasing the chances of bringing effective treatments to patients.
Integrating RWD and AI
Integration of RWD and AI enhances analytical approaches for successful indication selection. Advanced analytics help identify indications with a higher likelihood of success, reducing the risk of pursuing unproductive paths and optimizing resource allocation. This data-driven approach minimizes opportunity costs, accelerates new treatment delivery, and addresses unmet medical needs more efficiently.
Conclusion
The synergy between AI and RWD exhibits exponential growth, surpassing mere addition. As biopharma companies adopt data-driven, objective decision-making processes, the potential emerges to enhance drug development efficiency, mitigate risks, and expedite groundbreaking treatments to patients. This fusion of data and intelligence establishes a pathway for swifter, more targeted, and ultimately more efficacious drug development. With technological progress, the synergy between RWD and AI holds the potential to revolutionize the biopharmaceutical research landscape and promises a new era of precision medicine.